Understanding Artificial Intelligence: Concepts and Implications
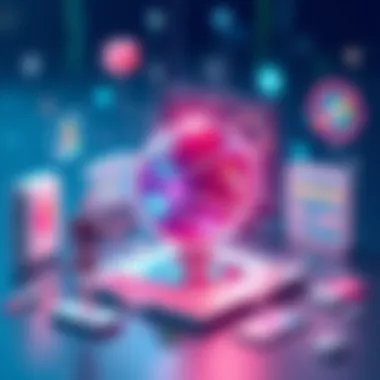
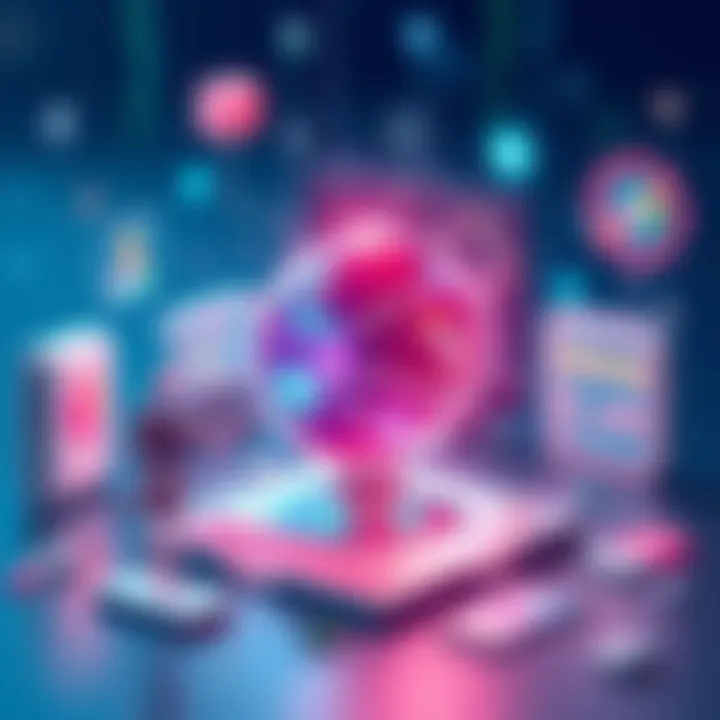
Intro
Artificial intelligence (AI) has become a cornerstone of modern technology, weaving its way into various industries and reshaping the landscape of how we interact with machines. This intricate field stretches far beyond the realm of mere automation; it encompasses data analysis, predictive modeling, natural language processing, and so much more. As technology continues to advance at breakneck speeds, it is crucial for IT professionals and tech enthusiasts alike to grasp the concepts that underpin AI and its practical applications.
The surge in AI's popularity isn't just about technical marvels; it raises pressing ethical questions. What are the implications of machines that can learn and adapt? How do we govern technologies that possess such power? This article embarks on a journey through the multifaceted world of AI, concentrating on key concepts, an array of real-world applications, and the ethical considerations that naturally follow.
Understanding AI means recognizing its potential to enhance industries ranging from healthcare to finance and transportation to entertainment. But it also requires a discussion on responsibilities—balancing innovation with accountability. Let’s delve deeper into its features, performance, and what the future might hold.
Features Overview
Key Specifications
Artificial intelligence can be broken down into different components that compose its architecture:
- Machine Learning (ML): Algorithms that enable systems to learn from data, identifying patterns and making decisions with minimal human intervention.
- Natural Language Processing (NLP): The capability of AI to understand, interpret, and generate human language, making interactions smoother and more intuitive.
- Computer Vision: This feature helps machines to interpret and understand visual information from the world, achieving tasks like image recognition and anomaly detection.
- Robotics Process Automation (RPA): The use of software to automate high-volume, repeatable tasks traditionally performed by humans.
Understanding these specifications provides a springboard into grasping what artificial intelligence can achieve within various sectors.
Unique Selling Points
What sets AI apart and why should organizations invest in it? Consider the following points:
- Increased Efficiency: AI systems can process vast amounts of information far faster than humans, leading to improved productivity.
- Enhanced Decision-Making: By leveraging data-driven insights, organizations can make more informed choices, minimizing risk and optimizing outcomes.
- Personalization: AI allows businesses to tailor their services and products to meet individual customer needs, thus enhancing user experience.
"The crux of AI's potential lies not just in automation but in its ability to liberate human creativity and innovation."
Performance Analysis
Benchmarking Results
In evaluating AI's effectiveness, one must look at specific performance metrics:
- Accuracy: How well does the AI model perform on its designated tasks?
- Speed: Time taken to process data and execute functions, crucial for applications like real-time analytics.
- Scalability: The ability to handle an increasing amount of work or expand to accommodate growth without compromising performance.
Recent studies reveal that AI can enhance operational efficiency by up to 30% in certain industries. Organizations that implement machine learning algorithms have noted substantial gains in accuracy and time savings, particularly in data-heavy environments like healthcare and finance.
Real-world Scenarios
To illustrate AI's impact, let's explore some practical applications:
- Healthcare: AI assists in diagnostics through image processing, predicting patient outcomes, and optimizing treatment plans. Companies like IBM Watson are leading the charge in this area.
- Finance: Algorithms detect fraudulent activities in real-time, while personalized financial advice is becoming the norm thanks to AI-driven analytics.
- Transportation: Self-driving cars, like those from Tesla, are revolutionizing how we think about mobility.
- Entertainment: Platforms like Netflix utilize AI to analyze viewer preferences and offer personalized recommendations.
Navigating the AI landscape is complex but vital. By understanding its features, performance, and real-world implications, IT professionals can harness the power of artificial intelligence and address the ethical questions that arise amidst this technological boom.
Intro to Artificial Intelligence
The topic of artificial intelligence (AI) holds significant weight in today's digital transformation landscape. From self-driving cars to intelligent personal assistants, understanding AI is not just an academic pursuit; it’s an essential pivot for IT professionals and tech enthusiasts alike. The rapid evolution of this technology invites both opportunities and challenges. Engaging with the tenets of AI now ensures that experts not only keep pace with advancements but also optimize their applications in diverse sectors.
A deeper insight into AI enables professionals to harness its potential effectively. For instance, consider health care—AI's ability to analyze vast datasets can profoundly enhance patient care and operational efficiency. Likewise, in finance, predictive algorithms can revolutionize risk assessment. Thus, the introduction of artificial intelligence not only highlights emerging technologies but also reframes our approach to solving real-world problems.
Furthermore, examining AI helps us scrutinize ethical implications and societal impacts. It’s not just about building smarter systems; it's equally about ensuring these systems operate fairly and transparently. In essence, the introduction to AI is about laying a foundation for an informed and responsible adoption of technologies that can shape our future.
Definition of Artificial Intelligence
Artificial Intelligence refers to the simulation of human intelligence in machines that are designed to think and act like humans. These intelligent systems are programmed to perform tasks such as learning, reasoning, problem-solving, and understanding language. In a nutshell, if a machine exhibits capabilities normally attributed to human intelligence, it can be classified as AI.
The complexity of AI is such that it encompasses a range of sub-disciplines—each contributing to the machine's ability to function intelligently. Key areas include machine learning, where systems learn from data, and natural language processing, which allows interaction in human language. The essence of AI is captured by the phrase: "mimicking human cognitive functions” and adapting these processes efficiently across numerous applications.
Brief History of AI Development
The origins of artificial intelligence trace back to classical philosophers who attempted to describe human thinking as a symbolic system. However, the groundwork for modern AI was laid in the mid-20th century. In 1956, the Dartmouth Conference marked the birth of AI as a field. Researchers like John McCarthy began to wield terms like "artificial intelligence" and ignited an era of exploration that was both promising and challenging.
Over the decades, AI cycled through phases of optimism and disillusionment. In the 1970s, significant advancements in expert systems heralded new applications in various industries. Yet, by the 1980s, funding dwindled amid unmet expectations, leading to what is known as the "AI winter." A resurgence began in the 21st century with breakthroughs in machine learning and vast improvements in computational power, giving rise to technologies such as deep learning and neural networks.
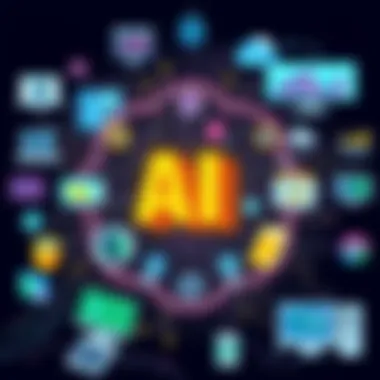
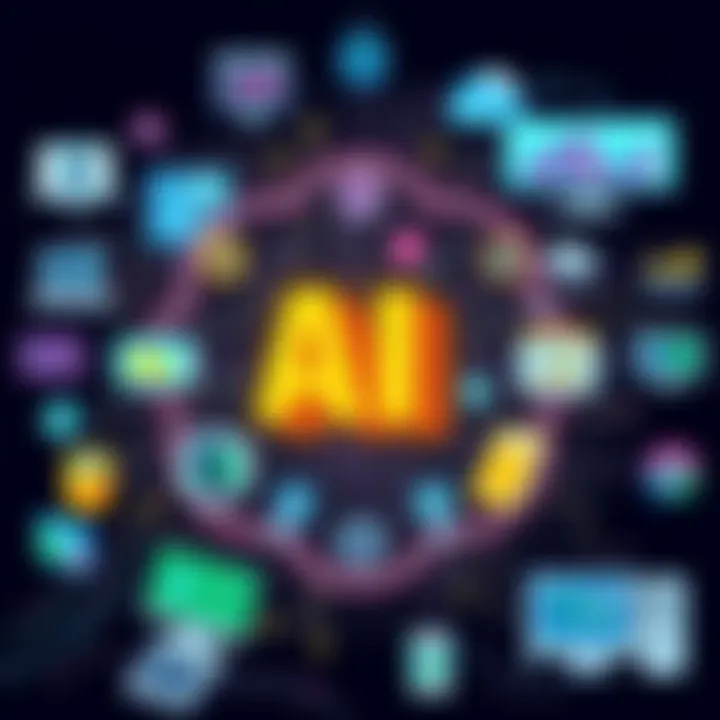
Today, AI is not a singular technology but a dynamic ensemble of methods and approaches that continues to evolve rapidly.
Key Terminologies in AI
An acquaintance with AI includes understanding its foundational concepts. Many terms are frequently encountered that carry specific meanings:
- Machine Learning (ML): A subset of AI where algorithms improve through experience. It involves providing data to enable machines to learn patterns without direct programming.
- Deep Learning: A further specialization of ML that uses neural networks with many layers to process data in complex ways, often applied in image and speech recognition.
- Natural Language Processing (NLP): A field focused on the interaction between computers and human (natural) languages, enabling understanding and generating text or spoken language.
- Neural Networks: Inspired by the human brain, these are networks of algorithms designed to recognize patterns.
These terms represent just a slice of the broader language of AI, but familiarity with them equips professionals to engage in deeper discussions about the technology's impact and its implications on society.
"Artificial Intelligence not only shapes our digital landscape but also challenges us to question our own perceptions of what it means to be intelligent."
Types of Artificial Intelligence
In the realm of artificial intelligence, discerning the various types of AI is crucial for understanding both its potentials and limitations. The categorization into weak AI and strong AI, alongside the distinctions between narrow AI and general AI, lays the groundwork for a comprehensive discussion about the technology's role in today's society. By grasping these differences, IT professionals and tech enthusiasts can better navigate the landscape of AI applications and the implications they carry.
Weak AI vs. Strong AI
Weak AI, often referred to as narrow AI, is designed to perform specific tasks without the ability to generalize from one context to another. Think of it as a skilled artisan: proficient in their craft, yet unable to step outside of that domain. For instance, voice assistants like Siri or Alexa are examples of weak AI. They excel at tasks such as answering questions or playing music but lack genuine comprehension or consciousness.
On the other hand, strong AI, also known as general AI, represents a more ambitious endeavor. This form aims to replicate human-like intelligence, possessing the ability to learn, understand, and adapt across a variety of domains. While still largely theoretical, strong AI presents an alluring vision of machines capable of reasoning, planning, and even possibly feeling.
"The challenge isn't just creating more intelligent machines, but creating ones that can truly understand us and our intricate world."
This duality of AI, illustrating the gap between task-specific machines and those aspiring to human-like cognition, raises profound questions about the future and ethical considerations surrounding technology.
Narrow AI Applications
Narrow AI is where the rubber meets the road, as a multitude of applications exist across diverse fields. These systems are tailored to address specific problems and have proved their worth in various industries. Here are some prominent areas where narrow AI shines:
- Healthcare: AI algorithms analyze medical images for signs of diseases, assisting radiologists in making more accurate diagnoses. Tools like Google's DeepMind have shown exceptional accuracy in detecting eye diseases from retinal scans.
- Finance: AI systems monitor transactions to detect fraudulent activities in real-time and provide investment recommendations using vast datasets. For example, JPMorgan's COiN platform can review and analyze legal documents with remarkable efficiency.
- Marketing: Targeted advertising heavily relies on narrow AI. Algorithms analyze consumer behavior to tailor content and product recommendations, leading to better engagement. Think Netflix’s recommendations—personalized, powerful, and incredibly effective.
Narrow AI has opened doors to innovation, but it also carries the weight of responsibility. Ensuring that these systems operate fairly and ethically is a crucial ongoing endeavor in the tech community.
General AI Concepts
While narrow AI captivates with practical applications, general AI continues to tantalize researchers and enthusiasts alike. Concepts associated with general AI touch upon whether machines can emulate emotional intelligence or possess the ability to reason abstractly. Many theorists debate the implications such capabilities would have on society, including the potential for machines to make moral judgments.
One significant concept in the discourse of general AI is the Turing Test, proposed by the mathematician and computer scientist Alan Turing. This test measures a machine's ability to exhibit intelligent behavior indistinguishable from that of a human. Despite the advancements in AI, passing the Turing Test remains a formidable challenge.
In contrast, the concept of superintelligence, where a machine surpasses human intelligence, remains a hot topic. Theoretical discussions often focus on the ethical frameworks necessary for overseeing such intelligence, underscoring the balance between innovation and moral responsibility.
Understanding these foundational concepts—a differentiation between weak and strong AI, and the distinction between narrow and general AI—illuminates the paths ahead. Navigating these pathways while acknowledging both the promises and perils involved is essential for those engaged in the technological evolution of artificial intelligence.
Core Technologies Behind AI
In the realm of artificial intelligence, understanding the core technologies is pivotal. These technologies act as the machinery behind the curtain, propelling AI into practical applications across various fields. This section delves into the essential elements that constitute AI, the inherent benefits they offer, and the considerations that come into play when adopting these technologies.
Machine Learning Fundamentals
Machine learning (ML) serves as a foundational layer of AI, enabling systems to learn from data and improve their performance over time without explicit programming. The primary aim of ML is to build algorithms that can make predictions or decisions based on input data. The techniques can range from basic linear regression to more sophisticated algorithms like decision trees and support vector machines.
- Supervised Learning: Here, algorithms learn from labeled datasets, allowing them to predict outcomes for unseen data. An example is spotting spam emails based on various features learned from a dataset of previous emails.
- Unsupervised Learning: This technique involves working with unlabelled data, where the model tries to find hidden patterns. Clustering algorithms, for instance, can categorize customers based on purchasing behavior without prior labels.
- Reinforcement Learning: Taking cues from behavioral psychology, this technique involves training models through feedback from their actions, like training a dog with rewards for good behavior.
A deep understanding of these fundamentals equips IT professionals with the know-how to develop robust AI solutions that can adapt and respond to real-world challenges.
Deep Learning and Neural Networks
Deep learning, a subset of machine learning, focuses on algorithms based on neural networks which are inspired by the human brain. These networks are composed of layers of interconnected nodes (neurons), each layer transforming the input data into something the next layer can use.
- Convolutional Neural Networks (CNNs): Predominantly used in image processing, CNNs excel at pattern recognition tasks. They are the backbone of technologies like facial recognition and autonomous vehicle cameras.
- Recurrent Neural Networks (RNNs): RNNs are tailored for sequential data, making them a perfect fit for tasks such as language modeling and time-series predictions.
The advantage of deep learning lies in its ability to automatically extract features from data without manual intervention, reducing human error in the model-building process. Still, these systems demand substantial computational power and large datasets for effective training.
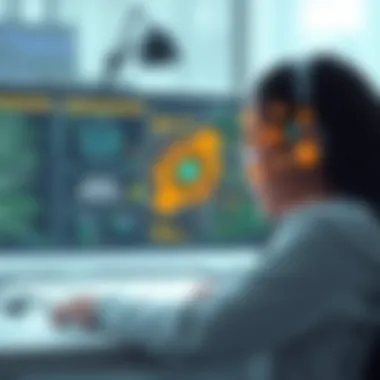
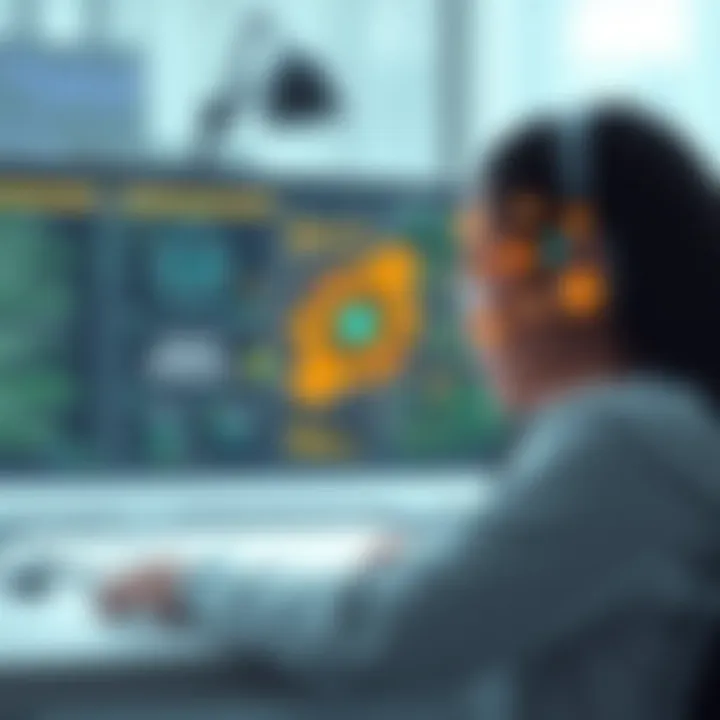
Natural Language Processing Techniques
Natural Language Processing (NLP) is pivotal for AI's interaction with human language. It encompasses everything from understanding spoken words to generating coherent text. NLP techniques are crucial in applications ranging from chatbots to translation services.
- Tokenization: This is the initial step where text is broken into individual words or tokens, making it easier to analyze.
- Sentiment Analysis: Through algorithms, NLP can assess the sentiment behind a piece of text, a feature valuable for businesses gauging customer satisfaction through reviews.
- Named Entity Recognition (NER): This identifies and classifies entities within text, like names of people, organizations, or locations.
NLP bridges the communication gap between humans and machines, making its role in the AI landscape ever more significant.
Robotics and Automation in AI
Robotics and automation are quintessential when discussing AI's real-world applications. These technologies integrate AI algorithms to perform tasks autonomously.
- Industrial Robots: In manufacturing, robots equipped with AI can adapt to varying production lines, significantly improving efficiency and safety.
- Service Robots: In sectors like healthcare, robots assist in surgeries or care. Their precision and consistency alleviate workloads from human staff.
- Drones: From delivering packages to agricultural monitoring, drones utilize AI to navigate autonomously and gather data.
The future of AI-driven robotics holds potential not just for increased efficiency but also raises questions about the ethical implications of such advances.
"The marriage of AI and robotics may not just reshape industries but redefine the boundaries of human capability."
Understanding these core technologies is vital for navigating the evolving landscape of AI. Each element plays a distinct role in shaping applications that address real-world problems, making the mastery of these fundamentals essential for professionals in the IT field.
Applications of Artificial Intelligence
Artificial Intelligence has seeped into many aspects of our daily lives, functioning silently yet effectively behind the scenes. Understanding the applications of AI not only offers insight into its relevance but also demonstrates its transformative capacity in different sectors. This section discusses noteworthy areas where AI is making waves, alongside key benefits and considerations associated with each domain.
AI in Healthcare
The healthcare industry stands as one of the most significant beneficiaries of AI integration. Technologies like machine learning and data analytics are reshaping diagnostics and patient care. AI systems can analyze vast datasets, producing insights that aid in early detection of ailments directly impacting patient outcomes. For instance, algorithms can scan medical images and detect anomalies that might escape even experienced radiologists’ eyes. This increases not just the speed but also the accuracy of diagnoses.
Nevertheless, there are concerns about data privacy and ethical treatment when using AI. Medical professionals must ensure that these systems are used responsibly, as misinterpretations or biases in AI can lead to unfortunate medical decisions.
AI in Finance and Banking
In finance, AI has become indispensable, streamlining processes and bolstering security. Applications include fraud detection systems that monitor transactions in real-time, flagging irregular patterns that could signify fraudulent activities. Machine learning models not only improve risk management but also personalize banking experiences for customers. Automated trading strategies powered by AI have changed the landscape of investment.
However, the reliance on these systems raises questions regarding transparency. Clients must be informed about how their data is used and how decisions affecting their finances are made. This demand for transparency maintains user trust, essential for the banking industry.
AI in Marketing and Customer Service
Marketing strategies are being revolutionized with AI as companies harness consumer data to craft targeted campaigns. Predictive analysis and customer segmentation stand out as vital tools in understanding market trends and consumer preferences. Bots powered by AI take customer service to another level, efficiently handling inquiries and complaints while maintaining a personal touch that enhances the customer experience.
Despite these advancements, it’s key to balance automation with human interaction. Over-reliance on chatbots, for example, can lead to customer frustration if issues grow too complex for automated systems to handle. Finding this balance is crucial for sustained success in customer relations.
AI in Transportation
The transportation sector is experiencing a paradigm shift thanks to AI. Self-driving cars and intelligent traffic management systems exemplify how AI is set to enhance safety and efficiency in urban mobility. Vehicles equipped with AI can analyze their surroundings and make real-time decisions, which might reduce accidents significantly.
As promising as this sounds, ethical dilemmas arise. The question of who is liable in an accident involving autonomous vehicles could lead to convoluted legal challenges. These uncertainties need addressing as the technology rapidly progresses.
AI in Education
Education is another field reaping the benefits of AI’s integration. Personalized learning platforms powered by AI adjust to individual students' learning paces and styles. Such customization can lead to better engagement and comprehension of topics that might have been otherwise challenging. Automated grading systems too free educators from monotonous tasks, allowing them to focus more on student interaction and curriculum development.
However, there are valid concerns regarding equity. If AI tools are not accessible to all students, the educational divide may widen instead of closing. Thus, ensuring everyone gets access to these advancements remains a crucial aspect that stakeholders need to consider.
The implications of AI applications are profound and call for careful consideration of ethical, social, and technical dimensions.
In summary, the applications of AI span various fields, showcasing its transformative power while also presenting challenges that require thorough examination. The ongoing evolution of these technologies indicates a future deeply intertwined with human tasks, calling for an adaptable mindset to navigate the ethical and practical dimensions of these innovations.
Ethical Implications of AI
The rapid advancement of artificial intelligence brings not only exciting possibilities but also serious ethical dilemmas. It's crucial to address ethical implications of AI in this discourse, especially as these technologies increasingly become intertwined with our daily lives. The key areas to explore include bias, privacy, and job displacement forecasts. Each element presents both challenges and opportunities that deserve careful consideration.
Bias and Fairness in AI Systems
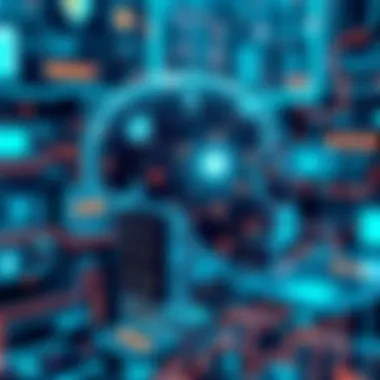
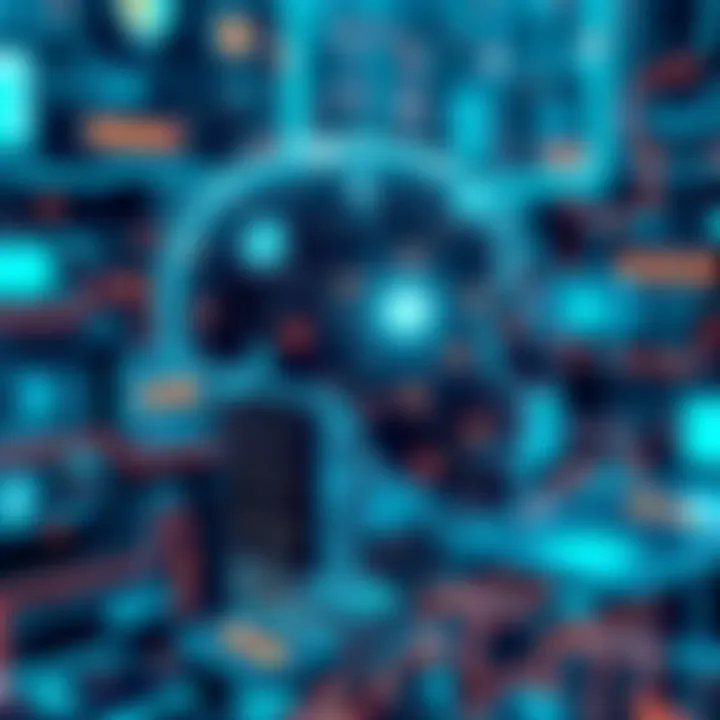
AI systems are only as good as the data they are trained on. Unfortunately, if that data is flawed, the outcomes will reflect those inaccuracies. Bias in AI happens when a system outputs unfair results, often due to historical inequalities embedded in the training data. For instance, facial recognition software has been known to misidentify people of color at alarmingly higher rates, raising concerns about discrimination.
To mitigate these biases, organizations must implement more robust testing and validation processes. Moreover, gathering diverse datasets is imperative to ensure the AI learns from a broad spectrum of experiences. Transparency in how these systems are developed also plays a significant role in fostering trust and accountability among users.
"The challenge lies not just in building intelligent systems, but also in being responsible stewards for their outcomes."
Privacy Concerns with AI Surveillance
With the advent of sophisticated surveillance technologies powered by AI, privacy has become a major topic of debate. Systems like facial recognition and predictive policing can lead to invasive monitoring of individuals. These technologies raise important questions: Who is watching you? How is your data being used, and for what purpose?
The widespread implementation of AI surveillance could strain civil liberties. Individuals may find themselves under constant watch without their consent, creating a chilling effect on free speech and personal freedom. To counteract these concerns, regulations and ethical guidelines must be established, balancing the benefits of enhanced security with the imperative of safeguarding individual rights.
Job Displacement and AI
The rise of AI also stirs fears of job displacement. Automation is increasingly taking over tasks once handled by humans, leading to concerns about unemployment and economic inequality. For example, roles in manufacturing, transportation, and customer service are particularly vulnerable, as machines can often perform these tasks more efficiently.
However, there's another side to this coin. Historically, technological advancements have also led to the creation of new jobs and industries. For instance, the expansion of AI has fostered demand for data scientists, AI ethicists, and other roles that didn't even exist a few years back. Upskilling and reskilling initiatives could be vital in preparing the workforce for this shift, ensuring that individuals adapt to an evolving job landscape.
In summary, while the ethical implications of AI present significant challenges, addressing these concerns can lead to more equitable and fair technologies. By prioritizing bias mitigation, data privacy, and workforce adaptation, the tech community can help shape an AI-driven future that benefits society as a whole.
The Future of Artificial Intelligence
The future of artificial intelligence holds crucial importance as it delves into not just what AI can accomplish, but also the broader implications for industries, societies, and individuals. As advancements in AI continue to unfold at a breakneck pace, understanding these changes is paramount for IT professionals and tech enthusiasts. This knowledge is essential in adapting to an evolving landscape that may redefine job roles, alter decision-making processes, and even influence global economies.
Emerging Trends in AI Research
Emerging trends in AI research are pivotal in charting the course for future developments and applications. Many researchers and organizations are turning their attention towards a few noteworthy areas:
- Explainable AI: As AI systems grow increasingly complex, the need for transparency becomes paramount. Explainable AI aims to make the mechanisms behind AI decisions understandable to users, which is crucial for building trust in automated systems.
- Federated Learning: This is a new area of research focusing on training machine learning models across decentralized devices. By doing so, it enhances data privacy, allowing user data to remain on devices rather than being uploaded to centralized servers.
- AI and Climate Change: Researchers are harnessing AI to combat climate change. Machine learning algorithms can optimize energy consumption, predict natural disasters, and offer insights into more sustainable practices.
Understanding these trends provides insight into how AI is being molded to tackle contemporary challenges, while also raising questions about ethics and accountability in technological deployments.
Challenges and Limitations of Current AI Models
Despite the advancements, there are notable challenges and limitations facing current AI models:
- Data Quality and Quantity: AI’s efficacy strongly hinges on data. Poor-quality or biased datasets can lead to flawed outcomes, perpetuating inequities or incorrect insights.
- Resource Intensive: Training AI models often requires immense computational resources and energy. This raises concerns about environmental sustainability and the accessibility of advanced AI capabilities to smaller organizations.
- Interpretability: Many AI systems act like "black boxes", making it difficult for stakeholders to understand how decisions are reached. This lack of explainability can hinder accountability.
These barriers can significantly shape the trajectory of AI, necessitating innovative solutions and fresh discussions around the ethical implications of using AI in sensitive areas.
The Role of AI in Shaping Societies
AI is poised to play a substantial role in shaping societies, influencing everything from employment to social structures. As this technology becomes integrated into daily life, its impact is multifaceted:
- Changing Workforce Dynamics: Automation threatens many jobs across various sectors, posing both a challenge and an opportunity for upskilling and reskilling initiatives.
- Social Interactions: AI-driven personal assistants and social media algorithms can affect how people communicate, share information, and build communities, often influencing public opinion.
- Healthcare Innovations: From predictive analytics in patient management to AI-powered diagnostic tools, the healthcare landscape is witnessing a metamorphosis that promises improved patient outcomes and efficiency.
"Understanding AI's influence in shaping societal infrastructure is essential for effective governance and ensuring technologies serve everyone equally."
Epilogue
The conclusion of this article serves as a vital component, encapsulating the intricate discussion on artificial intelligence. Not merely a wrap-up, it stitches together the threads of our exploration, guiding readers through the winds of AI's expansive landscape.
Recap of Key Points
Throughout our journey into AI, several key points have emerged:
- Foundational Concepts: We kicked off with a deep dive into what artificial intelligence truly means, uncovering its roots and historical development.
- Diverse Applications: AI's applications span wide and far—from healthcare innovations to advancements in finance, transportation, and education. The varied uses illustrate how this technology permeates everyday life.
- Ethical Considerations: The importance of ethics in AI cannot be overstated. Issues of bias, privacy, and job displacement are not just wrinkles to iron out; they are fundamental concerns that require ongoing dialogue and careful navigation.
- Future Outlook: Emerging trends signal the way forward, hinting at both vast opportunities and challenges that AI developers and users must confront in their pursuits.
AI as a Double-edged Sword
AI is like a double-edged sword, wielding the potential to cut through barriers of innovation while simultaneously posing significant risks. Its ability to process vast amounts of data can lead to breakthroughs in various fields, such as healthcare diagnostics or predictive analytics for business strategies. However, this very capability can also translate into ethical conundrums. Take, for instance, the algorithms used in hiring processes. If not crafted carefully, they risk perpetuating bias rather than eliminating it.
“AI stands at the crossroads of possibility and peril, demanding responsibility from those who wield it.”
As we navigate this landscape, it's crucial to remain vigilant about how AI affects society. The same technology that drives efficiency today could lead to widespread job loss tomorrow. Thus, the focus ought to be not only on advancing AI but also on framing its ethical use, ensuring it serves as a tool for progress rather than a catalyst for division.
Final Thoughts on AI's Evolution
The evolution of artificial intelligence is ongoing and complex. Each breakthrough, whether in machine learning or natural language processing, shapes the future landscape of technology. IT professionals and tech enthusiasts must consider the broader implications of their work. With each line of code written, we participate in a narrative that will define future societies.
In closing, a balanced perspective is essential. Embracing AI means we must commit not only to harnessing its capabilities but also to addressing the challenges it brings. As AI continues to evolve, let us not lose sight of the human aspect, ensuring that technology advances in a manner that aligns with the values we hold dear.